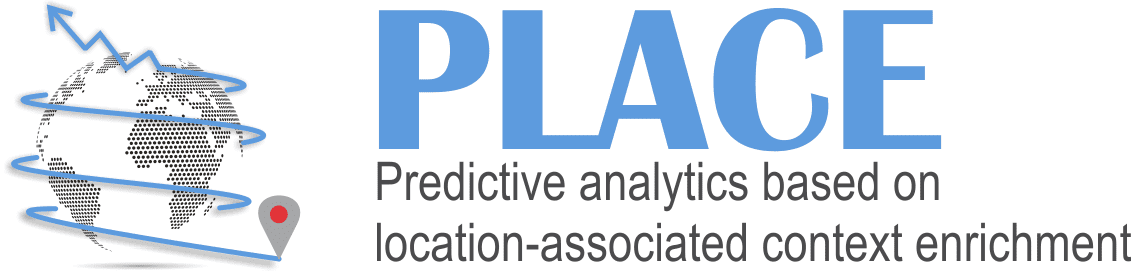
Period: 01. 05. 2017 – 01. 05. 2020
Funding SOURCE: ARIS
REFERENCE NR.: J2-8176
PROJECT PROGRAMME: basic research project
COORDINATORS AND CONTACTS: prof. dr. Domen Mongus
Project website: gemma.feri.um.si/PLACE/index.html
LINKEDIN: /
Predictive analytics based on Location-Associated Context Enrichment
Abstract:
In recent years, numerous aspects of decision-making have shifted noticeably towards data-driven paradigm, relying less and less on individuals’ intuition and more on data. This has resulted in an exponential growth of captured data, which is becoming increasingly more available and interoperable through open data initiatives (e.g. the Digital Single Market of the EC), open source software (such as GeoServer), and open standards (e.g. the OGC and INSPIRE Directives). Although contemporary methods are capable of aligning geospatial data from different information layers, assessing semantic features in a single or few aligned layers, and analysing raw data and/or extracted features, they often remain restricted to selected data types (e.g. hyperspectral and LiDAR or sensors connected through wireless networks), where the integration of domainspecific knowledge is relatively straightforward. Moreover, they do not incorporate data enrichment sufficiently, causing much of the data to be underexploited. As a result, many current state-of the-art methods are only capable of targeting situation assessment, while impact assessment is a characteristic of only a few domain specific decision support systems, where the contextual information is administered by users.
The PLACE (Predictive analytics based on Location-Associated Context Enrichment) project proposes to address these challenges by an in-depth study of the data fusion for context recognition, its structuring, and utilisation for improved predictive analytics in a geospatial domain. Within PLACE, we propose to advance on contemporary state-of-the-art by introducing context assessment as a separate process within data fusion. Conceptually, context shall be established above the assessment of geospatial entities, thus providing a new structured source of information to be utilised for improving high-level data fusion, i.e. situation and impact assessment. Additional advances shall be achieved by associating it to a process-centred framework, allowing for information generated by, e.g. simulations and regression models, to be fed back into the definition of geospatial entities. In this manner, we expect to enhance feature extraction (e.g. by improved assessment of semantic entities), provide additional (predicted) contextual information and, finally, improve reasoning in situation and impact assessment. The PLACE data fusion approach shall, thus, resemble a spiral model, capable of achieving “self-enrichment” of data during few iterations through the supporting processes. The latter include definition of geospatial entities (or Objects), feature extraction, context structuring, and predictive analytics.
The research activities shall be governed by two pilots, each serving as a test case which shall help us to concretise the developments and to design an appropriate methodology for hypothesis testing:
- Prediction of microclimate parameters using fusion of remote sensing (e.g. 3D LiDAR, meteorological, and satellite data) and in-situ measurements (e.g. eal-time measurements of humidity and temperature). The extracted contextual information will then be utilised for improved definition of fluid dynamics parameters in environmental simulations. Installed real-time sensors shall be used for accuracy assessment.
- Prediction of geomorphological changes caused by running water and floods, where structured contextual information extracted from remotely sensed data, historical flood maps, weather data, and existing maps of infrastructure shall be utilised within the regression models in order to estimate water erosion levels and the effects of floods on the terrain and infrastructure. Data from relevant past projects and supplementary field measurements shall be used for accuracy assessment.
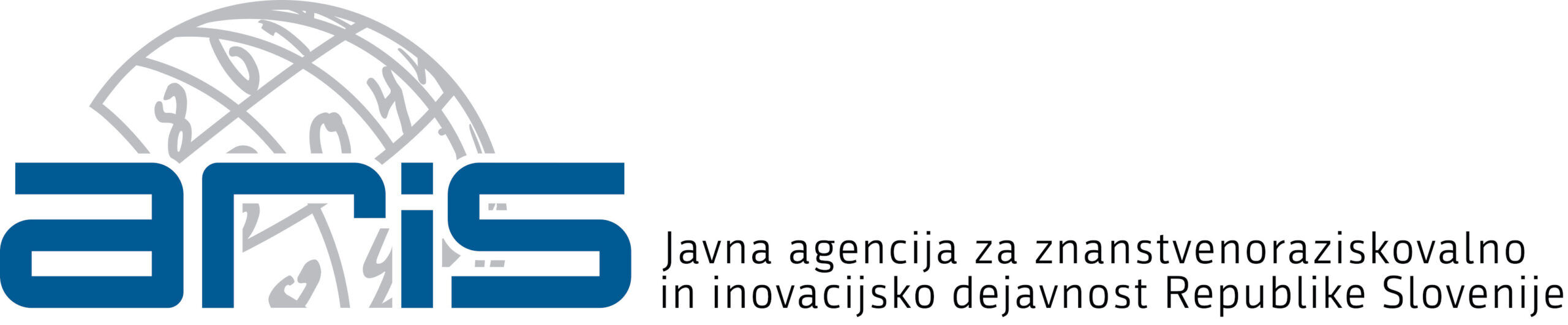